AI Seminar
Strategic Reasoning in Machine Learning, with Implications for Security and Fairness
Add to Google Calendar
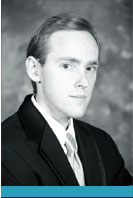
Abstract:
The practical success of machine learning has naturally led to a critical assessment of its underlying assumptions. One assumption that has received a great deal of scrutiny is that data is generated exogenously according to some fixed (albeit unknown) distribution. In other words, a typical model of data is as a mechanical, non-living entity, with no agency of its own. When people are involved in the process of generating data, however, human agency has a propensity to violate this assumption. In this talk, I will largely consider the case where people are strategic, manipulating information observable to learned models to their ends. Strategic manipulation of learning has a common mathematical abstraction in the literature: an actor changes their features, subject to a constraint on the magnitude of the change, to maximize prediction loss. I instantiate this in two settings: security and resource allocation. In the former, the strategic actor is an attacker who aims to achieve malicious goals (such as executing a malicious payload); in the latter, the actor is someone who simply wishes to obtain the resource, say, a loan. I will show that in a security setting, common intuition about the value of this simple threat model is inconsistent with evidence. In the case of resource allocation, I consider two issues that are qualitatively distinct from security: incentive compatibility and group fairness. In particular, I will discuss how one can achieve approximate incentive compatibility through auditing, and the phenomenon of fairness reversal that arises as a consequence of strategic manipulation of features. Finally, I will present the results of a \ human subjects experiment that studies perceptions of fairness of the information (features) used in a low-stakes simulated employment decisions, highlighting the importance of the role one plays (employer or prospective worker), as well as the differences between explicitly expressed and implicit sentiments.
Bio:
Yevgeniy Vorobeychik is a Professor of Computer Science & Engineering at Washington University in Saint Louis. Previously, he was an Assistant Professor of Computer Science at Vanderbilt University. Between 2008 and 2010 he was a post-doctoral research associate at the University of Pennsylvania Computer and Information Science department. He received Ph.D. (2008) and M.S.E. (2004) degrees in Computer Science and Engineering from the University of Michigan, and a B.S. degree in Computer Engineering from Northwestern University. His work focuses on game theoretic modeling of security and privacy, adversarial machine learning, algorithmic and behavioral game theory and incentive design, optimization, agent-based modeling, complex systems, network science, and epidemic control. Dr. Vorobeychik received an NSF CAREER award in 2017, and was invited to give an IJCAI-16 early career spotlight talk. He also received several Best Paper awards, including one of 2017 Best Papers in Health Informatics. He was nominated for the 2008 ACM Doctoral Dissertation Award and received honorable mention for the 2008 IFAAMAS Distinguished Dissertation Award.