Faculty Candidate Seminar
Unsupervised Learning for Large Scale Medical Image Analysis
Add to Google Calendar
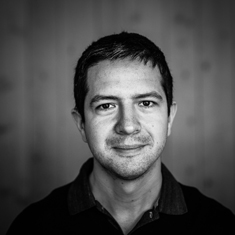
New approaches to the analysis of medical images can lead to new insights into complex diseases. In particular, there is a wealth of untapped clinical knowledge to be gained from the millions of diagnostic, low resolution clinical scans acquired every day as part of standard medical practice. In this talk, I will present machine learning techniques that can enable the use of clinical image collections in broad medical research studies. First, I define image imputation "“ the statistical inference of unobserved image anatomy. I describe an imputation method that exploits anatomical structure in collections of clinically-acquired low resolution scans, and learns to synthesize missing anatomy. This method enables subsequent studies not previously possible with clinical scans. Second, I present an unsupervised learning algorithm for deformable medical image registration that is up to 20,000 times faster than the current state of the art. This enables, for the first time, efficient processing of image collections comprised of tens of thousands of scans. Finally, I demonstrate analysis methods enabled by these algorithms that are currently being used in studies across twelve hospital sites to derive new insights about patients who have suffered a stroke
Adrian V. Dalca is a postdoctoral fellow at CSAIL, Massachusetts Institute of Technology, and Massachusetts General Hospital, Harvard Medical School. He obtained his PhD from CSAIL, MIT. His research focuses on probabilistic models and machine learning techniques that capture important relationships between medical images, clinical diagnoses, and other complex data. His work spans medical image analysis, computer vision, machine learning and computational biology. He received his BS and MS in Computer Science from the University of Toronto.