Dissertation Defense
Analysis of Situated Interactive Non-Expert Instruction of A Hierarchical Task to a Learning Robot
This event is free and open to the publicAdd to Google Calendar
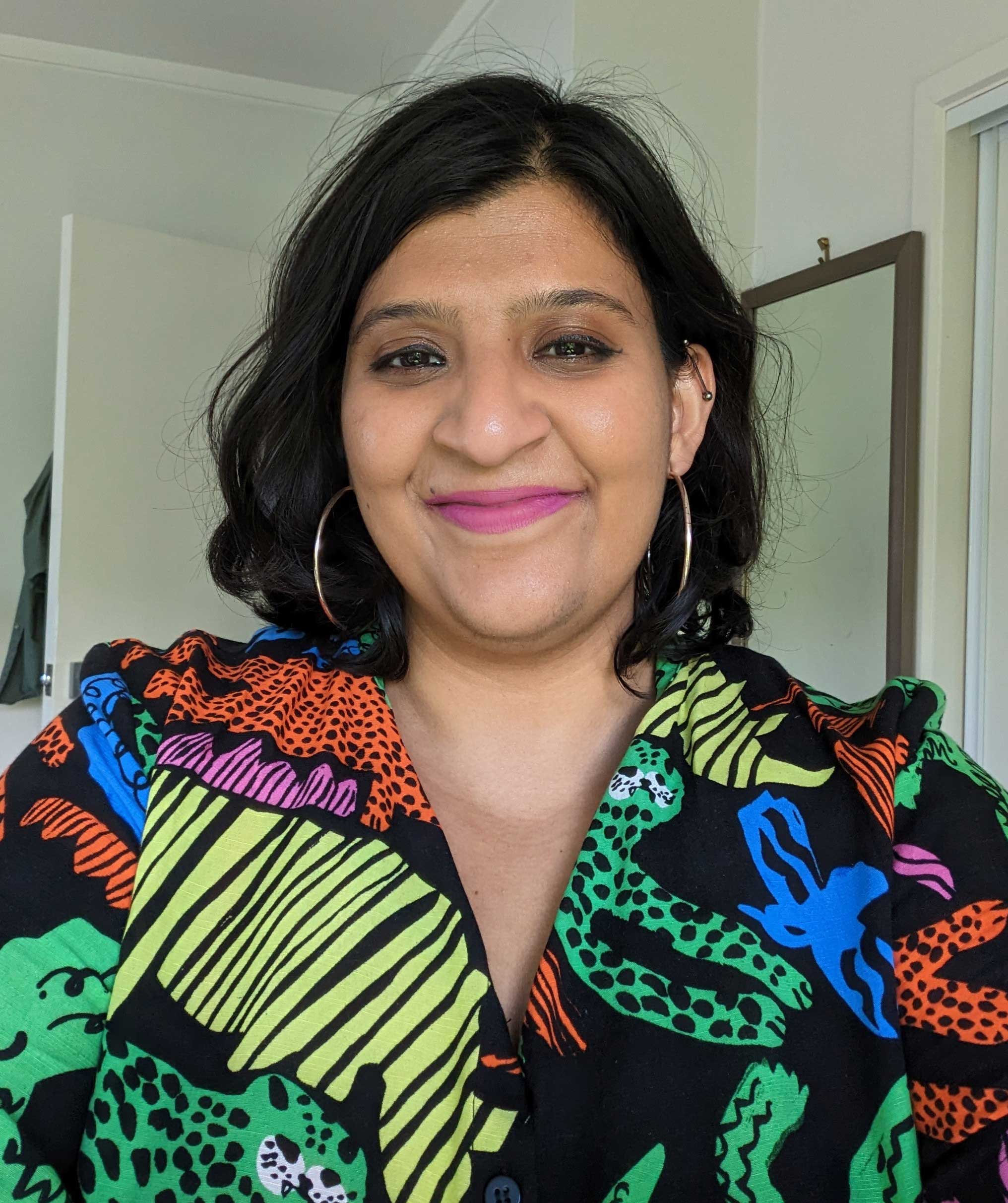
Hybrid Event: 3725 BBB / Zoom
Abstract: Interactive Task Learning (ITL) is an approach to designing robots that can learn tasks on the fly from human instruction and demonstration in a shared environment. ITL approaches until now have focused on extending a robot’s capabilities so that it can efficiently learn a wide variety of task components as a part of hierarchical tasks from an expert human instructor. However, the typical instructor is likely to be a non-expert who does not have a good mental model of the robot’s physical and mental capabilities and therefore is likely to face challenges during the teaching process. Our hypothesis is that we need to focus on building better robot learners that actively interact in a way to make the teaching process more accessible and efficient for the non-expert teacher. Towards this goal, we conducted a qualitative analysis of a human participant think-aloud study (N=14) where we asked participants to teach a multi-step hierarchical task of baking a pizza to an ITL robot. In this dissertation, we identify and characterize different aspects of the teaching processes used by non-expert teachers. We also identify the different types of knowledge they draw on during instruction including knowledge about the task, environment, interface, and the robot through these different sources. We identify relevant challenges encountered by non-experts during the teaching process which included difficulty in providing desired instructions, insufficient updates from the environment, and having incomplete and incorrect mental models of the robot that resulted in failure situations. We propose extensions to existing robot interaction approaches that can address these challenges and contribute to a more efficient and effective teaching experience for the non-expert.